Meet Julian Kee. A finance professional who became a data analyst without an IT background.
When Julian started working in finance, he discovered an interest in marketing. It was when he applied to move into a marketing position within the same company that he found out he enjoyed doing data analytics in marketing.
“I was interested to discover how customers think about our product and services,” Julian recalls.
The other thing Julian mentioned: was that when picking up data science, it can be overwhelming – just because you don’t know where to begin.
How to do data analytics without an IT background.
Julian is currently a data associate at 2X, a B2b marketing as a service (MaaS) firm with headquarters outside Philadelphia, PA, USA, and operations in Kuala Lumpur, Malaysia.
He works in the digital marketing industry, analyzing marketing campaigns from emails, social ads, user engagement on social media, performance, and more.
As he explains, a lot of the work he does involves using normal tools such as Excel, Google Data Studio, and platforms like ZoomInfo.com to identify business contacts.
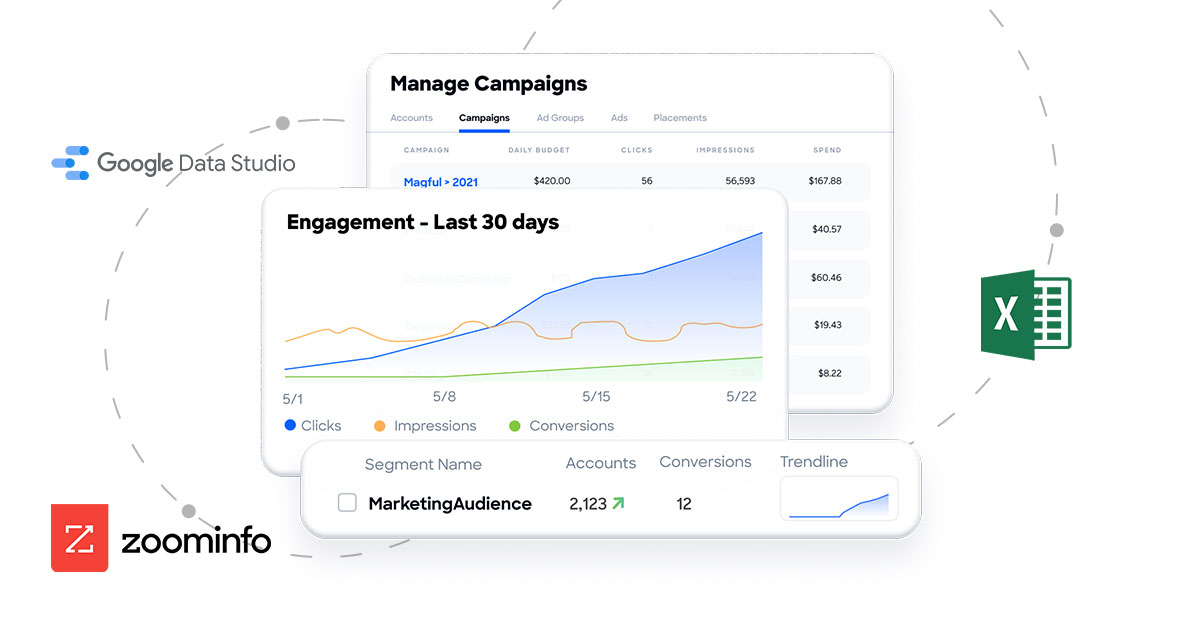
A large part of his work is to create analytics dashboards to show clients the most crucial information taken from multiple data sources – from the business and marketing campaigns.
All these tasks do not require you to have an IT background or a degree in computer science. There are many tools and platforms that will enable you to do efficient data analytics.
The bulk of analytical work is data cleaning.
True to what we have always taught in Data Science 360, the bulk of doing data analytics is data cleaning.
For Julian, this means having to do lots of data cleaning, especially on contact databases – for example, in cases where different users enter their contact information using a different format.
“Data cleaning is a very time-consuming job, but I think the most important thing is to find a quicker way. We usually use tools like Python for that. And it’s during moments when we couldn’t find a solution that we resort to manual data cleaning.” Julian says.
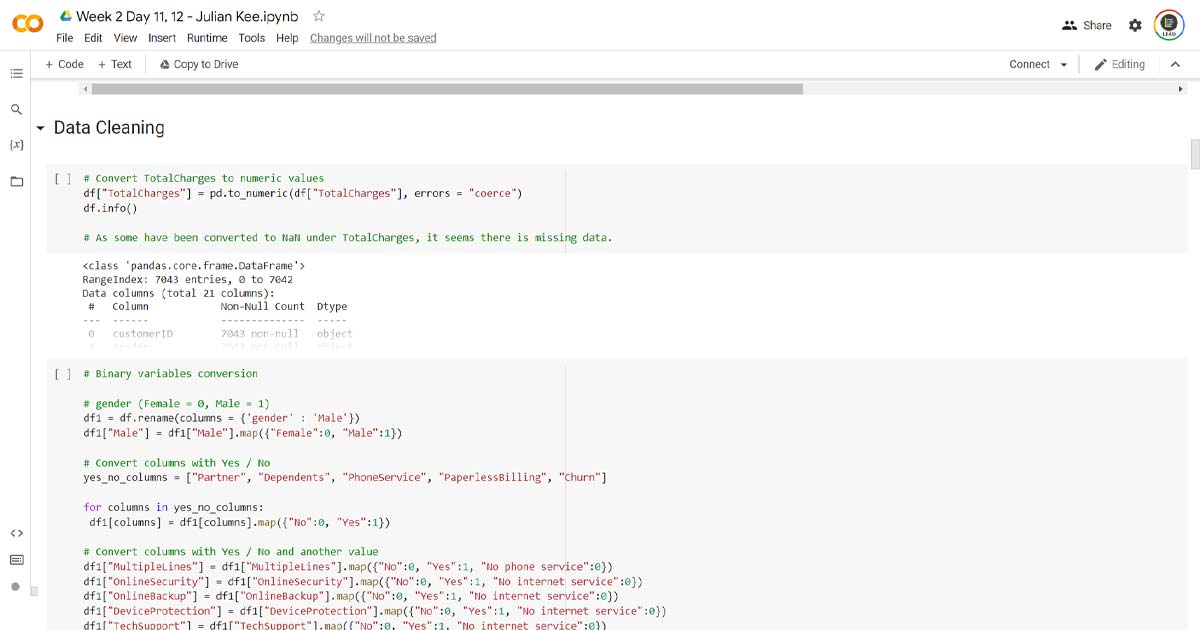
There are multiple ways to do data cleaning. A data analyst needs to identify the fastest, most efficient way of doing it.
It’s common to ‘not know’ where to start.
Julian did a business degree in university and first started working in finance. He had an interest in numbers and data but found his true interest in uncovering insights from marketing data over finance data.
“With data analytics in marketing, it is a lot about analyzing customer surveys and marketing campaigns, with a goal to understand how customers think about services and products”, Julian says.
“I didn’t learn automation until during my first job. I was asked to use Python, and that felt uncomfortable. It took me a while to get used to it before being comfortable to use it to analyze and automate stuff at work”, says Julian.
It’s quite common for beginners to not know where to start. With all the big words like ‘big data, ‘machine learning, and ‘artificial intelligence’ being thrown around in talks and on websites – it’s easy for a beginner to get paralyzed by overwhelm.
“That was my biggest challenge. Not knowing where to start, with no one to guide.” Julian said.
Not to mention time is also something you must factor in, especially if you hold a full-time job.
The best way to become a data analyst: “Expose yourself to things you don’t know”
Julian decided to join Data Science Uncut Bootcamp to learn different data analytics skillsets and data science problems.
For example, with his work in marketing analytics, a lot of what he does is measure quantitative data (data in the form of numbers). But during the Bootcamp, he was exposed to techniques to measure qualitative data (data that describes characteristics) such as sentiment analysis and machine learning.
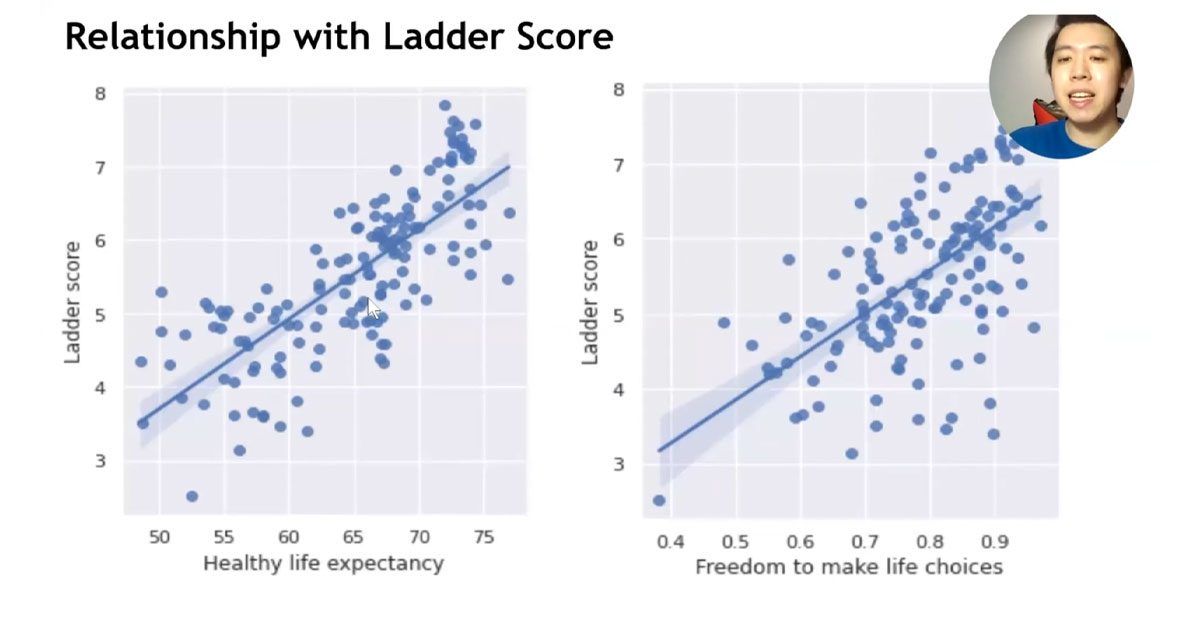
This enabled him to use different analysis strategies to solve different analytics problems.
Julian did not have an IT background as well. But when it comes to data science, you don’t have to be a computer science graduate before you can use programming tools like Python and R.
“When I studied statistics in uni, we were taught to use R programming. But not to use it as someone with an IT background. My professor told us that we didn’t have to call ourselves a programmer. We are just using a program to complete a task in data science” Julian recalls.
The best way to learn data science: Learn with the intention to teach.
One of the aspects that Julian appreciated most during the data science Bootcamp is the community.
“The community was really helpful. It was kinda like Stack Overflow, but only with people in Malaysia helping you. I see more chances of getting help, when you are getting it from people who are like yourself, in the same journey”, said Julian.
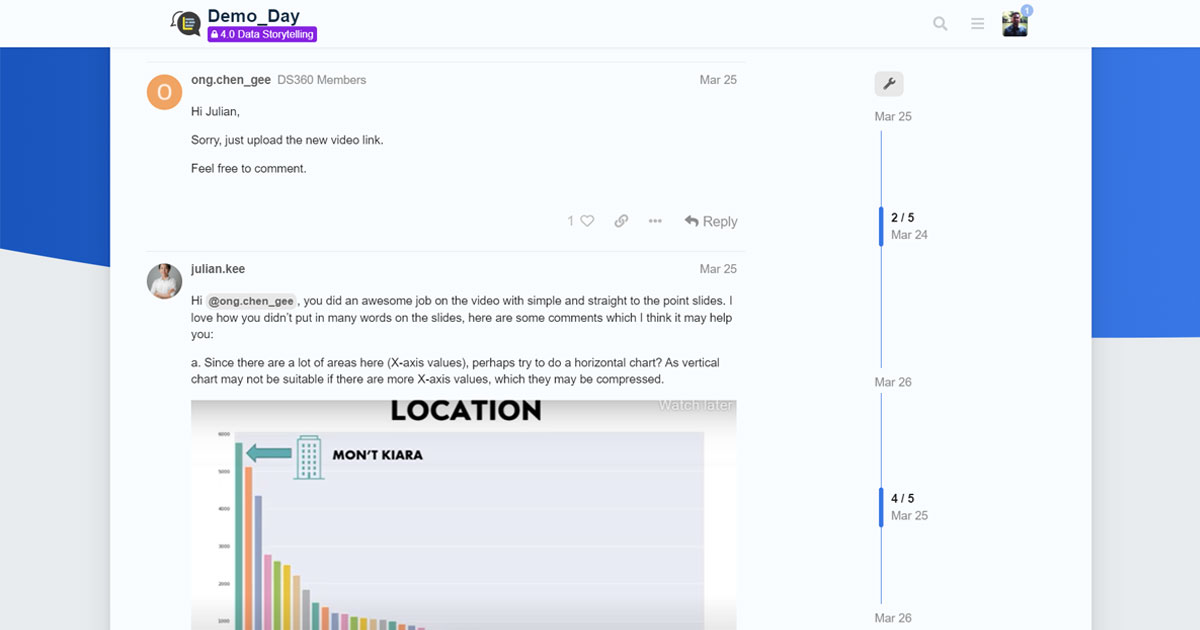
Julian mentions a lot about being able to self-learn and discover. With the student community, that worked together throughout the Bootcamp, created an opportunity for Julian to help the others as well.
As a result, he found that he learned faster. When you teach someone else, you get the chance of learning twice. First when you initially learned it, and second when you teach it.
He also mentions learning with the intention to teach. If you had to teach someone after learning something, you’d pay more attention and make sure to nail down important things – compared to if you would just learn it passively.
In fact, Julian doesn’t plan to do data science until the day he retires. His dream is to teach data science at a university.
“Throughout the Bootcamp, I think I loved the demo day the most. It was when I was able to see everyone.” Julian remarked.
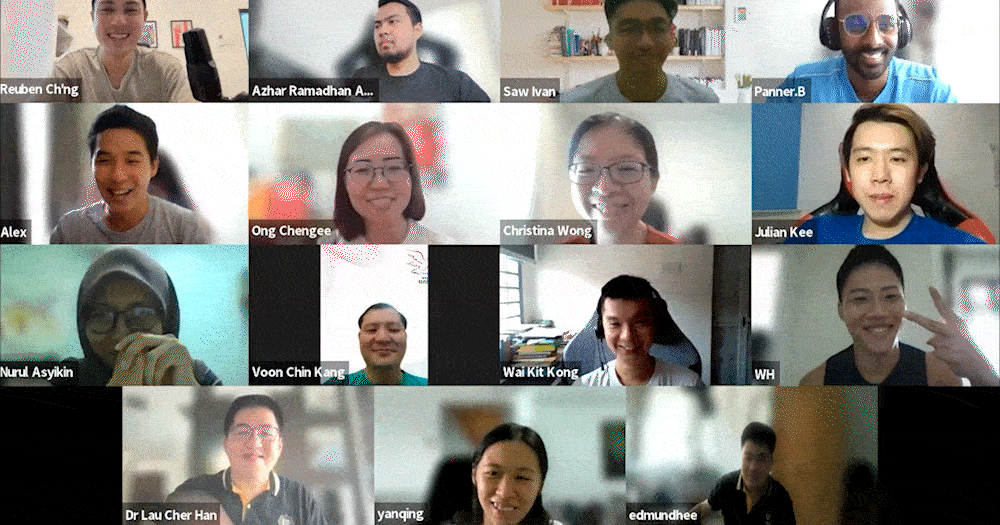
3 pieces of advice to become a data scientist in Malaysia.
When we asked Julian if he had any advice for people who wants to break into data science, here is what he said.
1 — Be Patient
“When you see a whole chunk of code, you’ll be intimidated. Especially if you don’t come from a tech background.” Julian said.
Most of the time, many people tell themselves they don’t stand a chance to become a data scientist, purely because of the fear of codes. But stay patient and give yourself the chance to go through codes line by line.
It’ll feel difficult at first because it is new to you. But eventually, it’ll begin to make sense – especially if you’re using an easy-to-learn programming language like Python.
2 – Know that you can’t remember everything.
A common misconception that beginners have is that they must memorize every Python code line, machine learning algorithm & analysis technique in data science.
“The truth is even very expert people, like Dr. Lau, googles for answers sometimes. It’s a normal thing for data scientists and programmers to do,” says Julian.
Sure, it’s important to have the fundamentals under your belt. But, don’t beat yourself up for not being able to memorize certain codes. This is where the beauty of the internet and communities comes in.
There’s always someone around the world who has faced the same problem as you and is willing to share a solution.
Remember, a good data analyst or data scientist is not someone who can memorize thousands of machine learning algorithms. A good data scientist is someone who can see clearly and solve problems.
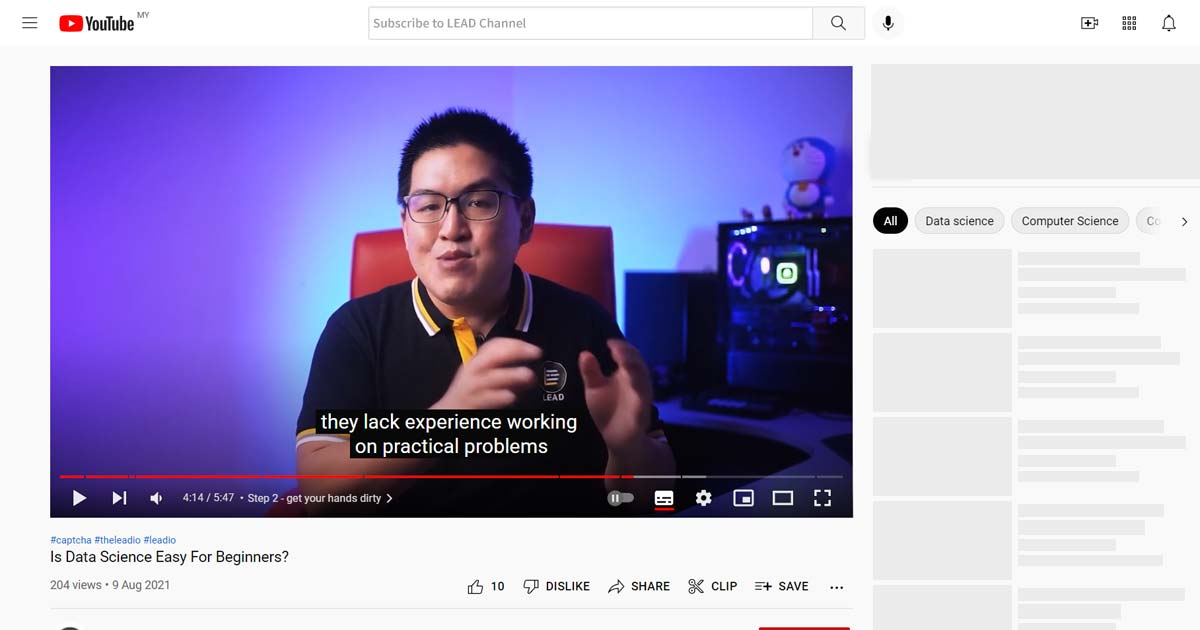
3 – Know your end goal.
Faced with a data science problem, you will be tempted to use fancy machine learning algorithms and charts to solve it. Working this way simply makes a project more complex than it needs to be.
Instead, Julian advises you to first know your end goal. Work backward from your end goal and find the steps that will take you there.
You can become a data scientist.
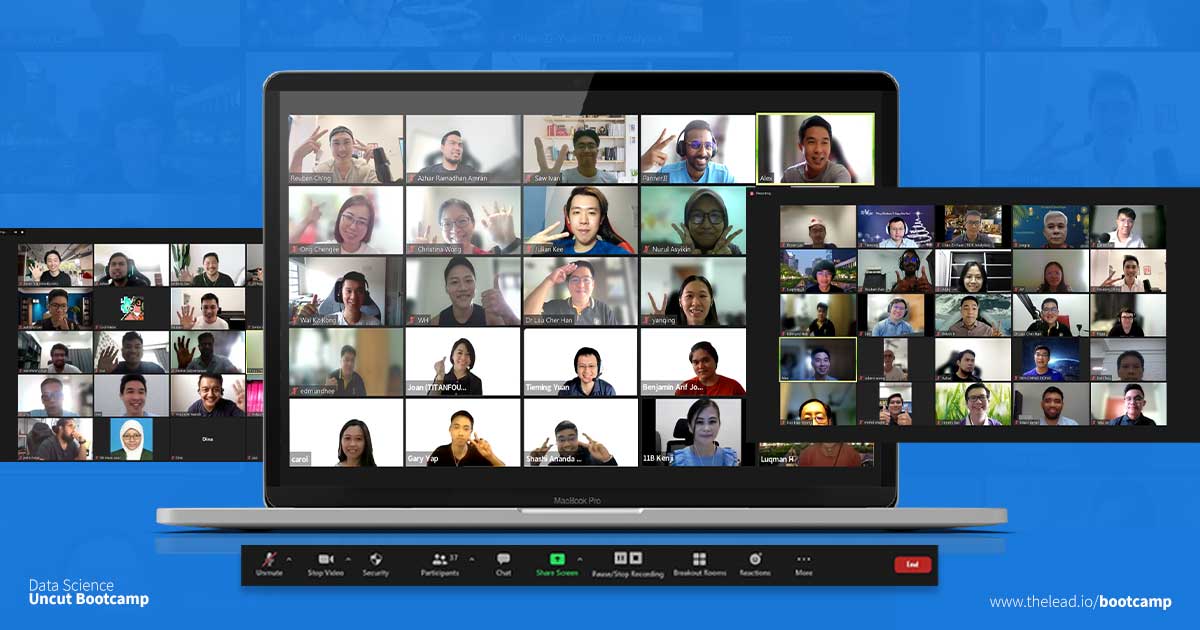
At LEAD, we’ve helped hundreds of people – Julian included, start and pursue a data science career. And we can help you too.
A great place to start is to enroll in our proven data science Bootcamp, which provides daily lessons and practice in practical data science techniques.
You’ll work together with a group of people to reach the end goal of becoming a job-ready data scientist.
Or if you’re still learning about the data science industry, watch our training on Breaking Into a Data Science Career for free. It covers everything you need to know about the data industry in Malaysia, including steps to build a career in it.
0 Comments