Here’s the biggest mistake companies make when collecting data:
Companies collect tons of data on their customers without knowing how to extract valuable information and make decision from them.
This is because it is easy to collect data. But oftentimes, we don’t always know the best possible method that gives us the most significant changes and valuable business insights. This includes asking the right key questions.
Which is why in this article, we had a chat with RJ Kevin, an accountant manager at Oddle who is passionate about data science, to find out the best ways we can process data, understand variable, factor, and relationship of data and users.
In this article, we will cover:
- The right approach to data analysis that gives us valuable insights
- How all companies, whether they’re long standing corporations or startups, are using slow, outdated techniques.
- How you can learn data science the most understandable, effective and fun way possible
1 – Data is Useless Without Insights
Because of the shift towards online business caused by COVID-19, we now realise how important an online presence can be to business sustainability. We also recognize how beneficial data science can be to maintain an online businesses
As a result, businesses all over the world are now adapting to this digitized landscape.
However, COVID-19 didn’t introduce anything new. It simply accelerated the ongoing movement of companies transitioning to become more data-driven.
It is no doubt, many companies are gathering as much data as they possibly can from individuals of the target audience to get the most accurate information of users relationship and decision making with their business.
This is because the information we get from data gives us a competitive edge – whether it’s from the simplest or advanced analytics.
Unfortunately, because people are rushing establish themselves online and implement data science elements into their company, many companies are missing the purpose behind data collection:
“Having data is great, but you must derive insights from it.”
That’s what RJ finds interesting about data. Even when companies have tons of data on their customers based on their RFM (Recency, Frequency and Monetary), they often don’t know how to deal with this data.
Your data is just going to be one big mess of information if you do not know the methods needed to extract insights.
To be able to bring value out of your data set, you first need to understand the objective or consideration behind your analysis.
For example: Customer segmentation is a marketing strategy that is personalized towards specific customers. The purpose behind customer segmentation is to discover which customers are to your brand and why.
What you should do with your data is to categorize them based on their RFM values and use classification models like K-clustering to group together customers who are the most similar to each other.
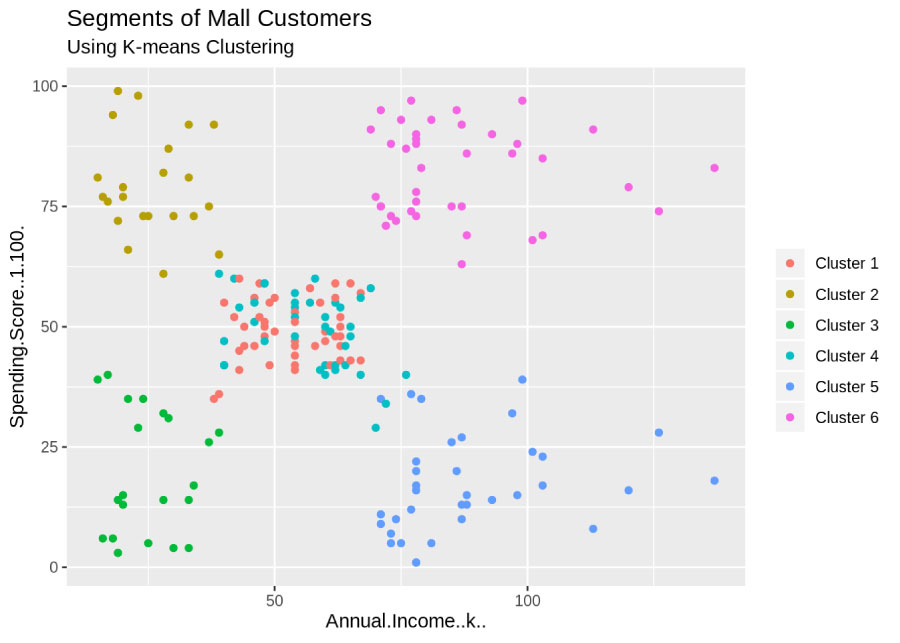
source: upGrad
After identifying your cluster, your objective is to invest in loyalty strategies for each group of customers to want to reach out to for more return sales.
By knowing your objective, you will also know the right methods to use to extract valuable data and even build useful model.
Going back to the example, using K-clustering categorizes customers quicker and more accurately compared to automated calculations on statistics in an excel sheet.
Learning the best data analysis process is the main reason why RJ decided to pick up data science.
2 – Even New Enterprises Use Old Data Analysis Techniques
Even as a high-tech startup that provides online menus and management systems for F&B businesses, RJ’s company Oddle was originally using excel sheets to analyse users data.
This is a common issue across all businesses who are starting to use data science.
Regardless of whether they are long standing corporations or recent startups, many companies are still using outdated, traditional methods to perform data analysis.
While it’s alright to use old techniques, they can be time consuming.
The insights brought forth by traditional techniques may also not be as significant as information brought in by newer, more efficient data science techniques.
In our fast-paced digital world today, we need to use time-efficient data analysis tools to meet the demands of our target audience.
After RJ had gotten the hang of using data science, he started teaching what he’d learnt to his fellow colleagues. After seeing how effective tools such as SQL codes, linear regression and logistic regression were at customer segmentation, they became invested in data science.
RJ has also been able to give more valuable and concrete advice in targeted marketing to his clients.
Smaller brands have an advantage in applying data science elements into their cooperation because they have yet to establish a concrete form of management, and have a mindset that is more open to learning new concepts in data science.
But as long as you’re willing to learn, any person can adopt data science into their business and grow.
3 – You Don’t Have to be a Genius to Learn Data Science
At first glance, the data science world seems complicated and overwhelming with many variables, factors, models, etc.
You’re not alone, many people including RJ often have this misconception.
Originally, RJ worked in sales for a year before becoming an account manager. The job scope required him to take up programming skills. RJ was fearful of data science because he had heard how hard programming was:
“I thought machine learning would be something only genius would take up.”
“But no, it’s not that. It’s beyond codes. Data Science not actually only about codes. You need to know a little bit of statistics, you need to know a little bit of communication, how you want to visualize that data to customers – so there [are] many aspects. It’s not just programming only.”
Once RJ entered the data science domain, he started getting the hang of it and found a passion in programming:
“I feel like programming is actually not hard to learn. After the Data Science 360 program, I realized it is actually pretty easy, and with all the support online, I think it is actually easy to self learn as well.”
His only regret was that he had not learnt it sooner. Having found his passion in programming, RJ sets aside an hour or two every weekend to further develop his data science skills in areas that are actually in his work.
When asked about how beginners can easily digest data science, RJ listed down 3 simple concepts:
1) Make your learning fun!
A common advice you’ll hear from instructors is to practice your programming skills by recreating case studies from Kaggle.
However, you do not necessarily need to pick and analyse the likelihood of diabetes or retail sales rates if you are not interested in that.
Get creative with your projects and find a fun topic to make a case study out of it!
Whenever RJ finds a topic that interests him on YouTube, such as the top 10 eateries in Petaling Jaya, RJ will go out of his way to research and find the available data, run it through his own models and share his findings with his friends.
Whenever you practice your skills with a case study, choose topics that either relate to your work or is something you are genuinely interested in; this will be your main motivator to keep on practicing!
2) Break down your studies into small pieces
The reason why data science is daunting is because it’s a big subject area with a lot of technical terms. You may feel like you need to learn everything in order to be able to understand it all.
However, trying to cram everything in your head will only cause you even more headache! It’s impossible to try to learn everything at once.
To better manage your learning process, break down your topics into little chunks and learn them at a short but frequent pace. You’ll find yourself learning and understanding data science much faster than learning a whole subject area in one go.
3) Be humble
When it comes to learning, many people often fall into the trap of feeling like they are an expert in data science after learning it for a few months…. Until the next big obstacle comes in their way.
It’s a common occurrence in the learning curve, and it’s good that you feel that burst of confidence.
However, if you really want to go far, you have to recognize that there’s so much more a person can learn and improve on. Learning is a continuous process. Once you have that mindset, you are thinking like a professional.
Final thoughts on learning data analysis
Anyone can process data, and any company can implement data science elements into their system.
But to make data work for you, you need to invest your time into learning the best data analysis techniques that relate to your work and your interest. You need to structure your learning in a way that best suits your needs.
Want to learn the best ways to approach data science? Sign up for our data science 360 classes here: https://thelead.io/data-science-360
0 Comments